Data Analytics | Healthcare | Disease Dectection
Data Analysis for Disease Detection at the Cellular Level: A Technological Approach
Notitia's Adelaide-based data analytics consultant, Jessica Molina Calabrese, explains how big data and data analysis methodologies has emerged as a powerful tool in biomedicine, particularly for analysing cells to identify potential diseases.
Data analysis has emerged as a powerful tool in biomedicine, particularly for analysing cells to identify potential diseases.
The vast amount of data generated by techniques like flow cytometry, DNA and RNA sequencing, and high-resolution microscopy offers enormous potential for uncovering patterns, biomarkers, and cellular characteristics associated with various diseases.
During her studies as a software engineer, Jessica Molina Calabrese, Notitia's Adelaide-based data analytics consultant, made a significant impact in early disease detection, volunteering her skills for a bioinformatics project that developed a program for the early detection of cancer cells. It required big data and data analysis methodologies to generate patterns in unhealthy cells.
"Leveraging these patterns, the systems can detect cancer cells with a high level of precision, achieving a success rate of 95%" Jessica said.
"I devised DNA tests to identify cancer cells, facilitating the prompt diagnosis of patients at the early stages of cancer.
"My research has made significant contributions to improving the efficacy of cancer detection, resulting in earlier diagnoses and improved treatment outcomes," she said.
In this article, Jessica explains how data analytics can assist in early disease detection to improve patient treatment outcomes.
1. Applications of Data Analysis in Cellular Analysis:
- Biomarker Identification: Data analysis can help identify biomarkers, which are molecules or cellular characteristics that are associated with a specific disease. These biomarkers can be used for early disease detection and monitoring disease progression.
- Cell Classification: Machine learning algorithms can classify cells into different categories, such as healthy cells, cancer cells, or infected cells. This classification can be crucial for diagnosing diseases and guiding treatment decisions.
- Disease Risk Prediction: Data-driven prediction models can be used to estimate an individual's risk of developing a disease based on their cellular characteristics. This information can be used for preventive measures and early intervention.
- Treatment Response Monitoring: Data analysis can be used to monitor the response of cells to treatment and evaluate the efficacy of drugs. This allows for personalised treatment adjustments to optimise patient outcomes.
2. Key Technologies for Data Analysis in Cellular Analysis:
- Machine Learning: This branch of artificial intelligence enables computer systems to learn without explicit programming. Machine learning algorithms are used for tasks like cell classification and disease risk prediction.
- Data Mining: This process involves extracting valuable information from large datasets. Data mining helps identify hidden patterns and trends in cellular data that might be missed by traditional analysis methods.
- Data Visualisation: The graphical representation of data facilitates its understanding and interpretation. Data visualisation tools help researchers visualise complex cellular data and communicate findings effectively.
- Cloud Computing: Cloud computing provides access to powerful computing resources over the internet, enabling efficient processing of large datasets generated by cellular analysis techniques.
3. DNA Sequencing to Data Table:
DNA sequencing technologies have revolutionised our ability to analyse genetic information. Transforming raw DNA sequence data into a structured, analyzable format involves several steps:
- DNA Extraction: Isolating DNA from a biological sample is the first step.
- Sequencing: Technologies like Next-Generation Sequencing (NGS) are used to read the DNA sequence.
- Base Calling: The sequencer identifies the individual bases (A, C, G, T) at each position in the DNA sequence.
- Data Processing: Raw sequence data is cleaned to remove errors and align sequences for further analysis.
- Variant Calling: This step identifies differences between the sequenced DNA and a reference genome, which might be potential disease-associated mutations.
- Data Tabulation: Finally, the processed data is organised into a structured table format for analysis.
4. Challenges and Opportunities in Data Analysis for Cellular Analysis:
- Data Integration: Integrating data from different sources, such as DNA sequencing, gene expression, and proteomics, remains a significant challenge. Combining data from various sources can provide a more comprehensive picture of cellular processes and disease mechanisms.
- Results Validation: Ensuring the reliability and clinical applicability of data analysis results through proper validation is crucial. Validation involves testing the findings in independent studies to confirm their accuracy.
- Model Interpretation: Interpreting complex machine learning models can be difficult, limiting their use in clinical decision-making. Researchers are developing new techniques to make these models more transparent and interpretable for healthcare professionals.
- Data Protection: Protecting patient data privacy is a paramount ethical and legal consideration. Secure data storage practices and anonymization techniques are essential to ensure patient privacy.
5. The Future of Data Analysis in Cellular Analysis:
Data analysis holds immense potential to revolutionise disease diagnosis, treatment, and prevention. As data analysis technologies continue to evolve and datasets grow larger, we can expect significant advancements in:
- Understanding diseases at the cellular level, leading to the discovery of new therapeutic targets.
- Developing personalised therapies tailored to an individual's specific cellular profile.
- Improving early disease detection through the identification of novel biomarkers.
6. Examples of Technological Applications in Data Analysis for Cellular Disease Detection:
- Development of Cell Analysis-Based Diagnostic Kits: These kits could enable rapid and accurate disease detection in primary care settings.
- Creation of Image Analysis Tools for Microscopy: These tools can assist pathologists in identifying cancer cells and other abnormalities with greater precision.
- Design of Simulation Models to Predict Treatment Response: These models could personalise treatment plans by predicting the effectiveness of different drugs for individual patients.
Data analysis is a powerful tool transforming the field of cellular analysis, opening doors to new possibilities for disease detection, diagnosis, and treatment. As technology continues to advance, we can expect even more innovative applications of data analysis in this crucial field of biomedicine.
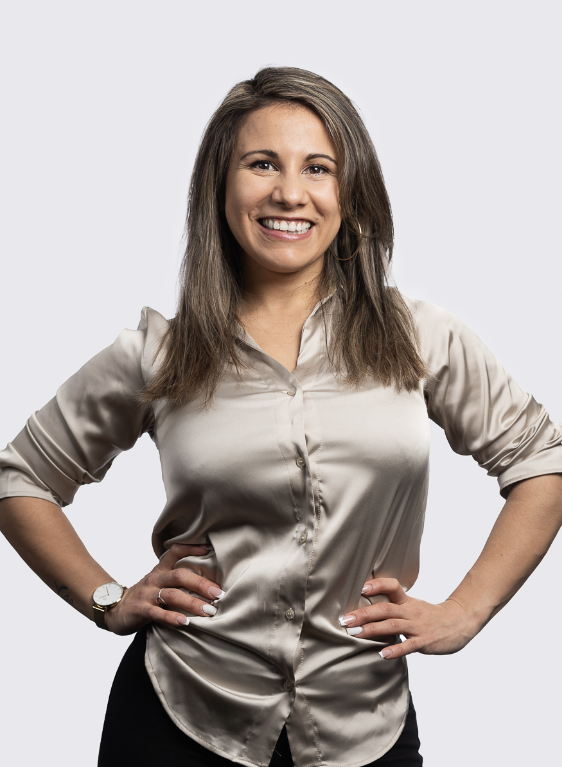
Jessica has spent 10 years delivering solutions in computer and data engineering. Beginning her career in the oil and gas sector, she has refined her skills internationally, working in Argentina, Boston, and Australia.
Known for her warm approach and solution-oriented mindset, Jess has extensive experience in digital transformation projects including on-premise and cloud deployment and migration. At Notitia she has worked alongside our clients including Foodbank Victoria and Barwon Water.
Get in touch with Jessica on Notitia's team page.